Next Generation 3D Understanding
ApplyProject Description
We are living in a 3D world. A broad range of critical applications such as autonomous driving, augmented reality, robotics, medical imaging, and drug discover rely on accurate representation of the three-dimensional data. While enormous efforts have been devoted to images and languages processing, it is still at an early stage to apply deep learning to 3D, despite their great research values. Here, we launch the project of next generation 3D understanding, and want to appeal more young talents like you to make this happen.
We are particularly interested in two topics of the next generation 3D understanding: 1) a large-scale pretrained foundation 3D understanding model; 2) a vision generalist model that connects 2D and 3D vision data such as images, point clouds, and RGB-D. For the first topic, you might have heard that the trillion-parameter AI language model Switch Transformer by Google Brain excels across nature language processing tasks, and you might also know about the recent model Imagen with over 2 billion parameters can produce Photorealistic images from texts. Both of them are great examples of the power of large-scale models. Unfortunately, in 3D understanding, even the largest well-known network is still with less than 100 million parameters. How to increase the scale of 3D models in order to further unveil the power of deep learning in 3D application is a promising research direction. For the second topic, as a human, we can understand vision data despite its modality (2D or 3D). It is a step towards general artificial intelligence in computer vision to propose a single model that is able to have all knowledge about vision including 2D (images, videos), 3D (point clouds, RGB-D). This is an interesting topic but is under explored in the community.
Our group at IVUL has put tremendous efforts and gained significant achievements in both topics. For the large-scale pretrained foundation model, our group is the first in the world that successfully trained a model with over 100 layers that achieved state-of-the-art performance in 2019 (DeepGCNs-ICCV19’). We broke our own record to 1000 layers in 2021 (GNN1000-ICML21’). Recently, we also propose scalable 3D networks with high inference speed in 2020s (ASSANet-NeurIPS21’, PointNeXt-arXiv22’). For the vision generalist model, our group has published impactful papers that involve understanding both view-images and point clouds (MVTN-ICCV21’, VointCloud-arXiv21’). Moreover, we have multiple ongoing projects in both directions. If you want to become a part in next generation 3D understanding, do not hesitate to join this project and achieve more with us!




About the
Researcher
Bernard Ghanem
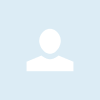
Desired Project Deliverables
(i) proposing a new large-scale foundation model for 3D understanding;
(ii) proposing self supervision techniques for training large-scale 3D models with limited data;
(iii) proposing novel generalist vision models that are able to tackle both 2D and 3D understanding;
(iv) proposing novel techniques for training this cross-modality generalist vision model.